Leo Pham
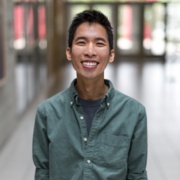
Streamflow forecasting is essential in water resource management and flood warnings. In the Western United States, streamflow is dominated by both winter precipitation and springtime snowmelt. Traditional physically-based and statistical models have been long used to predict changes in streamflow triggered by the onset of these events. In the past decade, advanced data-driven Machine Learning (ML) techniques have gained popularity as promising tools in modeling hydrological systems due to their comparative performance and cost-effective nature. In this study, we assess the predictive ability of Random Forests, a ML supervised algorithm that employs an ensemble of uncorrelated trees to yield prediction, in forecasting streamflow in large snowmelt/precipitation dominated river basins. Daily precipitation from PRISM AN81D, snow water equivalent (SWE) and temperature observations from Snow Telemetry (SNOTEL) Network, and discharge from US Geological Survey gages in the Pacific Northwest Watersheds are used for model training and validation. The accuracy of the model is compared against multiple linear regression predictions across four quantitative statistics: Coefficient of determination, Root mean squared error, Nashnash-Sutcliffe efficiency, and Kling-Gupta efficiency.